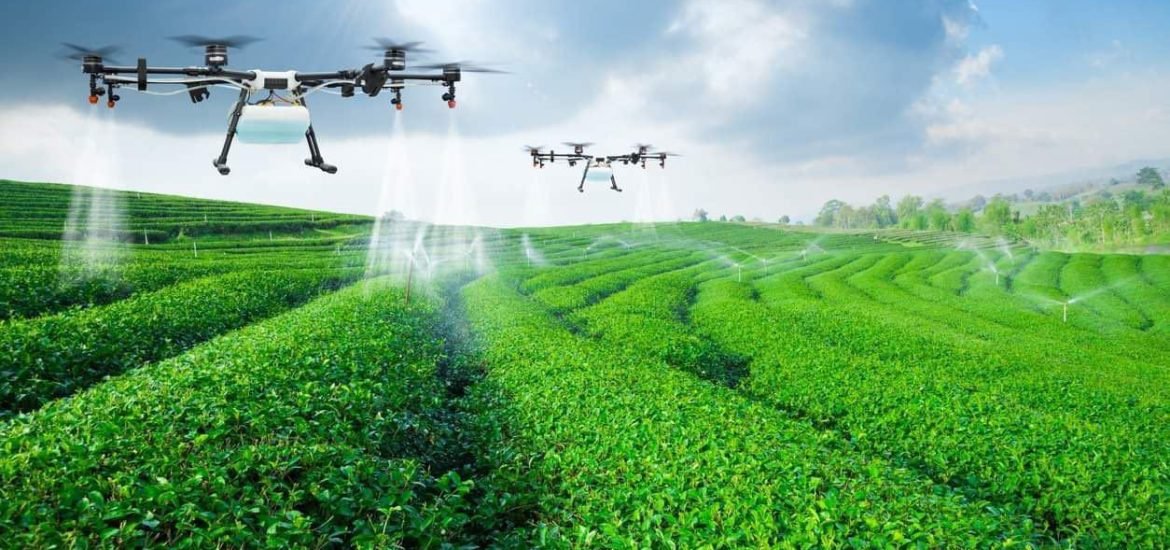
1. Is AI really needed in the agricultural world?
Modern agriculture has been at its 2.0 stage for a long time. Satellite or drone monitoring of crops, high-precision sensors to measure soil quality (humidification, salinity levels, and chemical composition), automated interventions to correct crop conditions; the agricultural world is neatly plotted with precise numerical measurements.
These new methods of data gathering and monitoring have made the agronomic world a major user of Big Data and the Internet of Things. What about AI?
Managing environmental constants would appear to fall under traditional data analysis techniques. Agricultural fields are like factories where each plant is a manufacturing unit, and managing them is similar to the maintenance tasks in the industrial manufacturing world: replenishing stocks of raw materials, repairing or replacing failed units, intervening with a particular product to bring the quality of production back within defined parameters.
The engineering practice for the living world appears to be an extension of mechanical and electronic engineering, an extreme case of production by sampling measurements and automated correction mechanisms.
In these circumstances, AI would not be necessary: the classic operational research process of activity optimization, standard statistical analysis, and the Internet of Things to increase the amount of data gathering would suffice. A factory is complex, but its operations are based on fully mechanisable processes without the need for AI. In fact, agriculture 2.0 has been using these technological advances for almost two decades.
The cycles of information measurement/analysis and prediction/decision and automated action can be summarized in the following two diagrams. The first describes the key points of collection and processing of agricultural data, and the second their main applications:
2. You can’t take the living out of the living world
The industrial use of agricultural data has been and continues to be of great importance. However, everyone knows that a plant is not just a simple mechanical or electronic production unit: a living plant is governed by far more complex processes. But understanding the essence of an entity clearly is one thing, and using it for human benefit is another.
For centuries steam engines were making use of thermodynamic phenomena much more complex than the physics of the time could describe. But by being located on the border of what could be controlled — stationary equilibrium states — trains or boats could be operated and were capable of enormous contributions to industrial development.
To paraphrase the key terms of Ilya Prigogine, the 19th century science of positivist physics believed that it had complete mastery over nature because it had understood all the detail of one branch of it: computable equilibrium states. Transient phases, which are highly unstable, were considered only as temporary disturbances which could be discounted, an ephemeral chaos falling between the lines of true physics, the physics that could be described with algebraic equations.
The whole history of modern physics — and the end of positivism — is the realization that the previous glorious trajectory of physics was just a vanishingly thin path across the vast expanse of knowledge and that the profound reality of phenomena was in the “discounted” zones: dissipative systems, non-equilibrium systems.
Traditional industry could simply remain within the narrow strip subject to “reason” and still achieve its ends and make use of nature to reach its goals. For more precision industries, or in dealing with extreme environments – the upper atmosphere, space, the great depths under land or sea – classic predictive models shatter under the complexity of natural phenomena: a physicist cannot continue simply to control a thermal machine from the outside, he has to enter the very furnace.
The same applies to agriculture. As soon as the living world is involved, incalculable phenomena have to be taken into account, for something we thought to be governed by an industrial logic. Between complete calculability and the unknown that can only be approached on an empirical basis, now lies an intermediate possibility: AI.
3. The limits of the factory analogy
The limits of the industrial exploitation of the land appear when we want to make detailed forecasts on crop development, an essential requirement for economic productivity.
An initial problem arises with regard to the different dimensions of an aerial shot, whether it is carried out by satellite, drone or any other means. The main parameters of the image capture are the spatial resolution, the time interval of the shots, and the spectral signature of the soil being imaged, which needs to be representative of its usage and composition.
It is very difficult to obtain high-performance images covering all these three dimensions at once, because the high resolution, the range, and the spectral accuracy require equipment that cannot be used too frequently.
While the use of drones represents a real improvement compared to satellites in terms of the frequency of shots, they do not currently permit heavy instrumentation for image capture, nor do they provide any significant capacity for storing and transmitting data.
The shots are, of course, supplemented by measurements on the ground, including the composition and temperature of the soil at different depths, as well as the condition of the plants, based on sampling.
This framework of data allows for a high degree of precision, but at the same time reveals the scale of difference between an agricultural field and a factory.
If we are comparing plants to a production unit, then these are really very special units, the like of which no factory has ever seen anywhere in the world.
If we were to continue the analogy, we would have to imagine totally interdependent production units, subject to the effects of propagation, osmosis, and symbiosis between each other. There is nothing like that in a factory, which is a set of units which are independent of each other, or simply connected by the transmission of a few signals, supervised by a central program.
On the contrary, a field is a set of units that are permanently interdependent, highly connected, prone to effects of contagion, self-organized by laws of reinforcement exerted among themselves, and not managed by a central control unit. This is more evident in a forest, where the concept of an ecosystem is well accepted and understood, including trade-offs between plants and insects.
Ears and stalks neatly arranged next to each other may seem like independent units in a factory. This is true only at first glance and for a short period of time. If you want to make detailed forecasts over a period exceeding a week, you have to think of the field in terms of a body as a whole, which hosts a range of propagation effects between the different units.
This post is also available in: FR (FR)
I m currently studying